
Have song lyrics gotten less complex?
The evolution of lyrical diversity of popular music over time
By: Isaac Piraino

Since the dawn of Youtube, there has been constant bickering about how complex and magical music from the previous generation used to be when compared to the allegedly simple music that dominates our airwaves today. New music is often criticized for being repetitive and basic, while old-school music is praised for its poetic richness. The new music vs. the old music lyrics question is so pervasive that Jay-Z cleverly showed us his self-awareness when he rapped:
“If skills sold, truth be told, I'd probably be lyrically Talib Kweli/ Truthfully I wanna rhyme like Common Sense/ But I did 5 mill' – I ain't been rhyming like Common since.” -“Moment of Clarity,” Nov. 14, 2003
For those that don't listen to rap, Talib Kweli and Common are acclaimed lyricists, and Jay-Z's point is that, in order to make more money with music, you gotta keep it simple. Today, we are going to see exactly how true that is. We will be exploring the following questions:
- How do we measure an artist’s lyrical diversity?
- Are simple songs more popular?
- How has this evolved over time?
Numerically measuring lexical diversity
Unfortunately, comparing complexity is, well, complex. In the field of applied linguistics, there are several mathematical measurements that can be used to understand written text. Simple approaches involve counting the amount of unique words (N.D.W. = Number of Different Words), and more sophisticated approaches measure the amount of unique words compared to the amount of total words. Linguistics defines the latter as lexical diversity, and experts don't all agree on exactly how to calculate it. The crudest way is to take the amount of unique words and divide by the amount of total words (T.T.R. = Type-Token Ratio).
A practical calculation of T.T.R. should ensure that we don't count different forms of the same word as separate occurrences. This is achieved through a process called lemmatization, which converts words to their root form (e.g. car, cars, car's , cars' are all converted to “car” and are not counted as 4 unique words). Calculating T.T.R. also involves making sure that unimportant function words like “the,” “in,” and “a” are not counted. Lastly, T.T.R. should be used on text lengths of at least 100 words to have any meaning. Ignoring the length requirement, I've included a poorly constructed gif to illustrate how we find the T.T.R. of the sample sentence “This blog took an inordinate amount of time to make; I hope this makes money.” :
The problem with T.T.R. is that, as your text size gets bigger, you are more likely to repeat words, causing T.T.R. to go down. This makes T.T.R. unsuitable for comparing song lyrics that vary in size. A better attempt at capturing lyrical diversity involves measuring how few times T.T.R. drops below a pre-defined threshold as you read the text. This produces an index that is no longer skewed by the length. It is known as the Measure of Textual Lexical Diversity (M.T.L.D.) and I promise it's the last acronym I'm going to be throwing your way. I won't go into the weeds of how to calculate M.T.L.D., so if you want the full explanation, this paper sums it up nicely, and provides more strengths and weaknesses of other methods used to calculate lexical diversity. The example sentence we used earlier to demonstrate how T.T.R. is calculated has an M.T.L.D. score of 15.0, and that's not useful for a few reasons. First off, like T.T.R., M.T.L.D. should only be used on text samples of over 100 words. Secondly, and more importantly, we need to see the M.T.L.D. of other pieces of text and see how they compare to get some perspective. To answer the question of perspective, I scraped hundreds of thousands of song lyrics from the internet. I tried to specifically target songs from popular artists, so I scraped the Billboard 200 Top Album Chart dating back to 1963. Then, I scraped every song from every artist that ever appeared on the Billboard 200. I ended up with 450k songs with over 100 words. I calculated their M.T.L.D. scores and graphed the distribution, which you can see below.
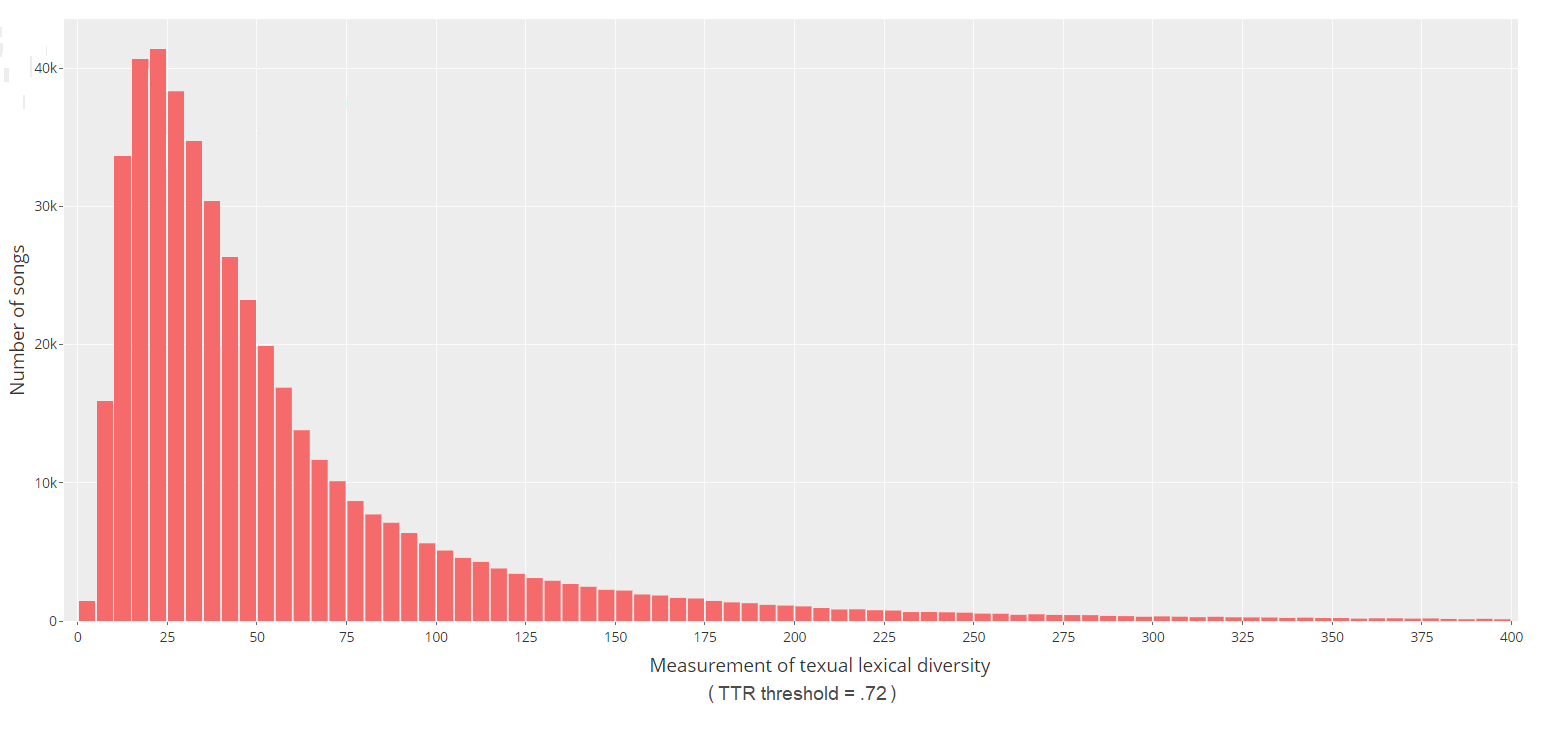
We can immediately see that most of our songs have an M.T.L.D. that falls between a range of 10-75, with the median score across the entire dataset being 38.48. What you don't see are 2,500 songs that had a M.T.L.D. of over 400. These high scoring songs never go over the threshold T.T.R., and the M.T.L.D. algorithm rewards songs the longer they go without ever exceeding it. This reward can be quite dramatic in the top scoring songs. In other words, if you want to get an off the charts M.T.L.D. score, keep word repetition above the threshold T.T.R. for as long as possible. To make this even more concrete, I think it would be better if we look at the M.T.L.D. scores of some individual examples to get a better idea of how different scores compare against each other.
- “Katrina’s Fair,” Natalie Merchant: 3,265.91 (highest score)
- “Alphabet Aerobics,” Blackalicious: 1075.76
- Henry V, opening chorus, Shakespeare: 377.26
- “Moment of Clarity”, Jay-Z: 132.38
- “Isis,” Bob Dylan: 70.47
- “One,” Metallica: 57.54
- “Perfect Illusion,” Lady Gaga: 18.75
- “Gucci Gang,” Lil Pump: 12.74
- “Around the World,” Daft Punk: 3.00
A list of all the songs and their respective scores can be found for free here. If you're a data scientist, into pop culture data, or just plain curious and would like to do your own lyrical analysis, I have the entire dataset of all the lyrics available for download for free here. Now, let's revisit what Jay-Z said in “Moment of Clarity”. In the song, Jay alleges that he has simplified his lyrics for the sake of popularity. We can examine exactly how his M.T.L.D. has changed since he released that song on Nov 14, 2003. As for popularity, I got the data straight from the horse’s mouth. I went to Jay-Z's music streaming website, Tidal.com, and discovered that their backend system has a datapoint called ‘popularity’. It seems to be a number from 1 to 100 where 100 is most popular. It is undocumented, and doesn't seem to be shown anywhere in their UI. After looking at some of the data points, it does seem to reflect reality, but since we don't know how it's calculated, it can only be our best guess.
Visually, this distribution doesn't present a clear winner, but when we crunch the numbers, his average popularity increased 11% from 49.8 to 56.0 while his average M.T.L.D. decreased 13% from 89.74 to 78.04. This proves that Jay-Z did simplify his vocabulary while increasing in popularity. However, this doesn't answer the general question about the overall simplicity of all popular music and how it has changed over time. In order to accomplish this, I took the average of each month’s M.T.L.D. score for the Billboard 200 Top Albums for every month since 1980.
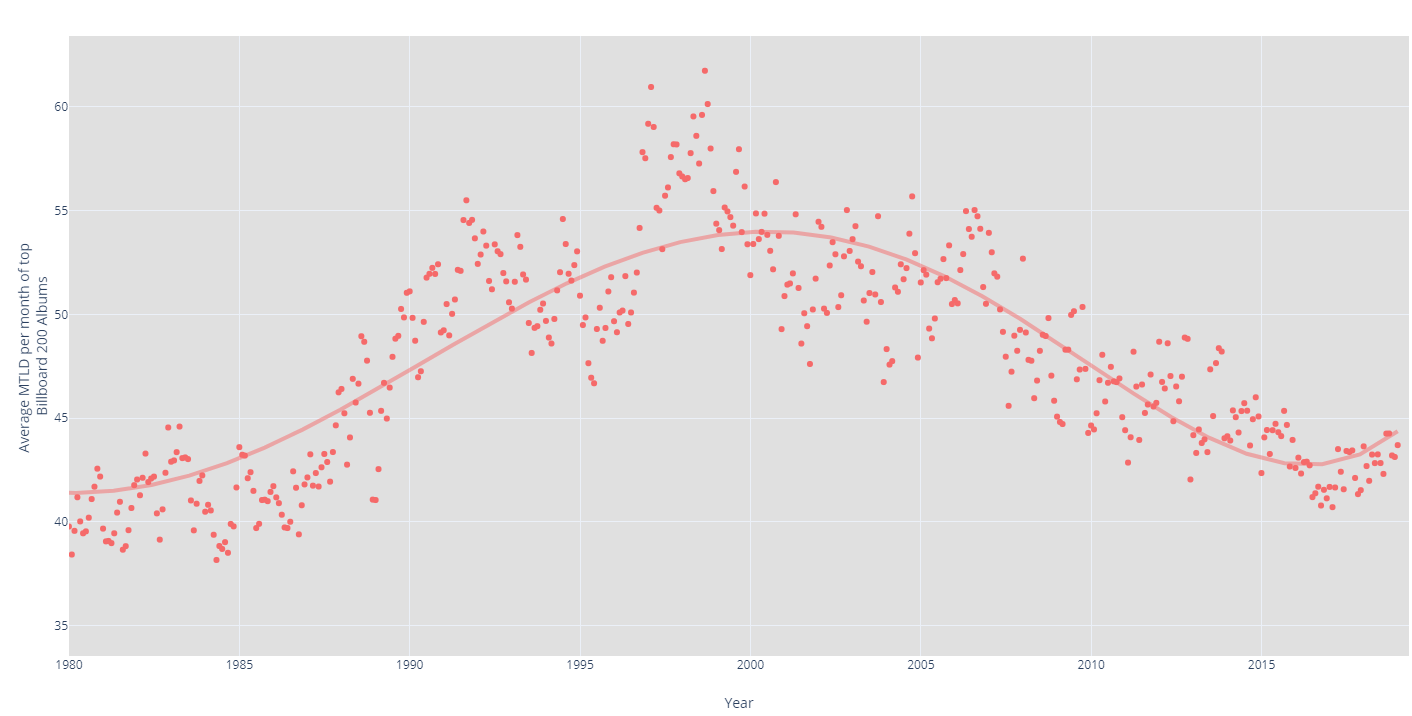
This paints an interesting picture. We can see that M.T.L.D. scores went up until the late 90s and then started going back down from then to the present day. My theory is that the gradual decrease in the popularity of rock music and increase in popularity of hip-hop explains the upward trend to the end of the 90s. Rock music, although complex in different ways, usually has a more simple vocuabulary than its lyrically dense hip-hop counterpart. My theory for why it went back down after the 90s is that hip-hop has slowly been transforming into pop music in combination with the rise in popularity of EDM (electronic dance music). Music critics and the pioneers of 80s/90s hip-hop have weighed in on the issue of the transformation of hip-hop into "hip-pop" . As for EDM, it is lyrically the most simple genre out there. EDM typically has a handful of catchphrases that are repeated over and over again, which results in the lowest M.T.L.D. scores-- ones that can reach the single digits!
We've seen how popular music's lyrical diversity has evolved over time, but we still haven't seen how it compares to less popular music. Luckily, the dataset I've put together has songs that made it to the Billboard 200, as well as songs from the same artist that didn't make the cut. This gives us an excellent point of reference for making our comparison.
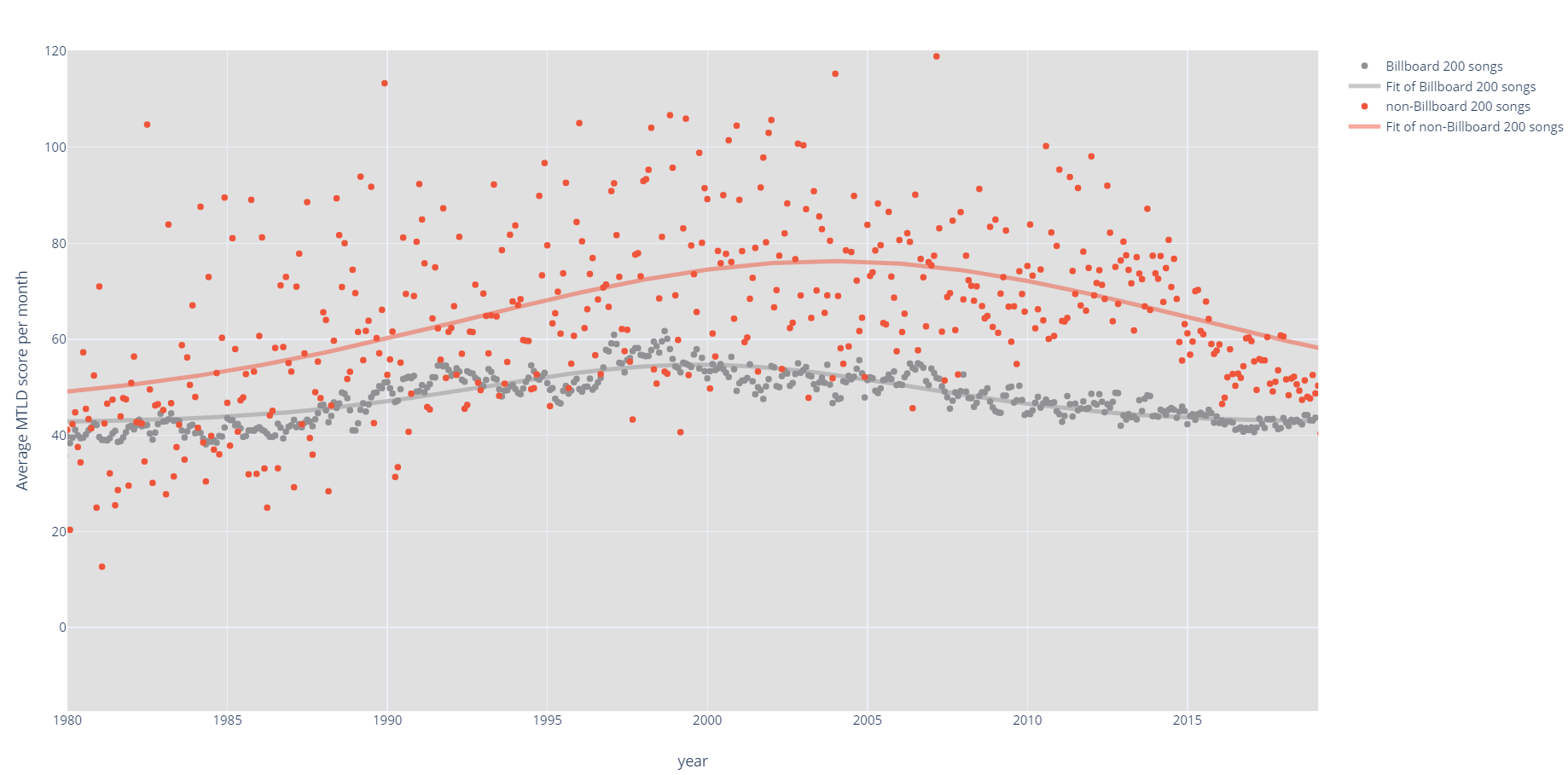
Now we can finally answer if popular songs are more lyrically diverse. So if your friend asks you, “Hey buddy, you think the average M.T.L.D. of unpopular music is higher than popular music?”, you should probably get some new friends because that's a strangely specific way to ask a question. Then, you can answer his question with: “Well, buddy, according to an article I read on the Internet, the answer is yes, by a whopping 18%!!”
Conclusion
We learned that music has had a non-straightforward evolution in lyrical diversity, and we've also found evidence for proving popular music is typically less lyrically diverse. I think it's important to note that the overall complexity and quality of music cannot be captured by a simple metric, if at all. My analysis specifically focused on the diversity of vocabulary, and even then, you can be profound with few words. At the same time, your message can lack lyrical substance while having a varied vocabulary. My only intention was to provide some insight on one particular facet of this multi-dimensional issue. This was my first crack at doing anything natural language processing related, and I am equal parts fascinated and astounded at the complexity of how language works. There's so much context and nuance in understanding language, and my next post will be a little more rigorous in trying to capture this. Thanks for reading!